

Srinivas Balagopal
Abstract
Srinivas is currently a sophomore at Pinewood High School in Los Altos Hills, CA
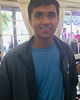
Urban air pollution levels pose serious health hazards. Almost 80% of global urban populations live in deleterious urban microclimates. The nonlinear dynamics of urban air pollutant dispersions are influenced by topographical and anthropogenic factors, which impede both the efficacy of existing deterministic pollutant forecasting models and the ability to predict pollutant impacts on the populations living in microclimates. Therefore, the purpose of this study was to use nonlinear correlation analysis and historical meteorological and pollutant data to construct a localized and pollutant-specific nonlinear forecasting model using Recurrent Neural Networks for an urban microclimate in San Jose, California. The study also uses the predictions to refine pollutant impact on the local Air Quality Index (AQI) calculation, by utilizing an Analytical Hierarchical Process (AHP) framework that mathematically weighs the effects of the criteria air pollutants specific to this microclimate. The predictive model’s results indicated 95% accuracy in forecasting hourly changes to pollutant concentrations. The AHP was used to develop an aggregated AQI that represented pollutant prevalence in the urban microclimate. The study conclusively proved that pollutant concentrations in San Jose were caused primarily by anthropogenic factors but were also influenced by unique climate and topographical factors. While the study’s results are limited to San Jose, the methodology can be applied to other urban microclimates because the model is predicated on specific topographical and anthropogenic characteristics. Pollutant remediation must be addressed at a municipal level, by analyzing the impact of pollutants on specific microclimates and on the local population.